AI Assistants
AI assistants are software tools that use AI technologies to perform tasks, answer questions and assist with decision-making to improve efficiency and productivity.
Boost efficiency and customer satisfaction with AI assistants
AI assistants are making our lives more efficient and convenient. Leaping beyond traditional chatbots, they understand natural language, process commands and perform tasks effectively. By leveraging machine learning and natural language processing, they learn from interactions and improve over time, enabling businesses to automate tasks, enhance efficiency and provide personalized support, ultimately boosting productivity and customer satisfaction.
Healthcare
Scheduling, reminders and basic symptom assessments.
Retail
Handling customer inquiries, personalizing shopping and managing inventory.
Hospitality
Answering guest questions, recommending activities and booking appointments.
Finance
Monitoring transactions and alerting users to potential fraud.
Manufacturing
Predicting equipment failures and scheduling maintenance.
Education
Creating customized learning plans and tracking progress.
AI assistants built for you
WWT creates custom AI assistants tailored to meet diverse business needs, from streamlining RFP processes and enhancing project management to automating HR functions and providing intelligent chatbots for support and communication.
Check out these demo videos and discover more about what AI assistants can do.
WWT-developed AI assistants
WWT built Atom Ai to support our entire organization by integrating diverse data sources and generating detailed insights.
Building on Atom Ai's foundational capabilities, WWT's RFP Assistant streamlines the proposal qualification and response process.
We developed Document Assistant to simplify how organizations handle and summarize large volumes of documents.
See how we did it
Get a behind-the-scenes look at our internal GenAI development process in these multi-part reports from WWT Research.
While use cases will vary depending on the industry, AI assistants can help organizations realize benefits such as:
Increased efficiency
Automating repetitive tasks allows employees to focus on more strategic activities.
Enhanced productivity
Handling routine inquiries and tasks frees up time for employees to work on higher-value projects.
Improved customer service
Providing instant responses to customer queries improves response times and customer satisfaction.
Cost savings
Automating tasks can reduce operational costs by minimizing the need for human intervention.
24/7 availability
Operating around the clock provides support and services outside of regular business hours.
Data-driven insights
Analyzing large volumes of data provides actionable insights and recommendations.
Scalability
Handling a growing number of tasks and interactions without a proportional increase in costs.
Consistency
Providing consistent responses and services reduces the risk of human error.
Personalization
Tailoring interactions based on user data and preferences enhances the user experience.
Enhanced decision-making
Processing and analyzing data quickly aids in faster and more informed decision-making.
Comprehensive AI Assistant development
Utilizing the diverse AI Proving Ground lab environments and following this detailed methodology, WWT tailors AI Assistants to meet your unique needs, delivering engaging and reliable user experiences.
Client consultation: We begin by understanding your specific needs and the context in which the AI Assistant will be used. This involves detailed discussions to identify the most compelling scenarios relevant to your industry and audience.
Scenario planning: Based on the consultation, we develop potential use cases. For example, in the healthcare industry, we might explore scenarios where the AI Assistant helps with scheduling, reminders and basic symptom assessments.
Technology selection: Leveraging advanced platforms, we define the infrastructure architecture and design the AI Assistant to include features like natural language processing (NLP), machine learning (ML) and real-time data processing. This allows the AI Assistant to interact seamlessly and naturally with users.
Customization: We tailor the AI Assistant's functionalities, responses and behavior to align with your brand identity and user expectations.
Understanding data sources: Collaborating to identify and integrate relevant datasets, ensuring compatibility with the AI Assistant.
Outcome alignment: We map specific functionality, like NLP capabilities or workflow automations, to your goals and industry requirements.
Initial development: We create a prototype for you to review. This prototype serves as a preliminary version to gather feedback and make necessary adjustments.
Client feedback: You are presented with the prototype for detailed feedback. This iterative process helps the final version meet your requirements and expectations.
Creative problem-solving: Throughout the development process, we address any technical challenges that may arise.
Additional functionality: We enhance the prototype with the functionality and features that you request so the completed AI Assistant meets all your specific needs. This may include:
Natural language processing: For seamless communication.
Workflow automation: Streamlining repetitive tasks, like scheduling or notifications.
Integration with existing tools: Ensuring compatibility with CRMs, databases or third-party apps.
Real-time analytics: Offering actionable insights from user interactions.
Custom branding: Tailoring the Assistant's tone and visuals to reflect your identity.
Quality assurance: We conduct extensive testing to confirm the AI Assistant's responses are accurate, interactions are seamless and the overall user experience is polished.
Collaborative effort: Our development team works collaboratively to identify and resolve any issues, ensuring the AI Assistant performs flawlessly.
System architecture: After successful testing, the AI Assistant is deployed in your environment—in the cloud, at the edge or on-premises—with a robust system architecture.
Training and documentation: We provide comprehensive training and documentation, including video and written instructions, to help your team effectively operate and demonstrate your AI Assistant's capabilities.
AI Proving Ground environments
The AI Proving Ground contains multiple pre-defined multi-OEM architectures and almost limitless custom configurations. This enables building, testing, training and validation of all the components of enterprise AI solutions.
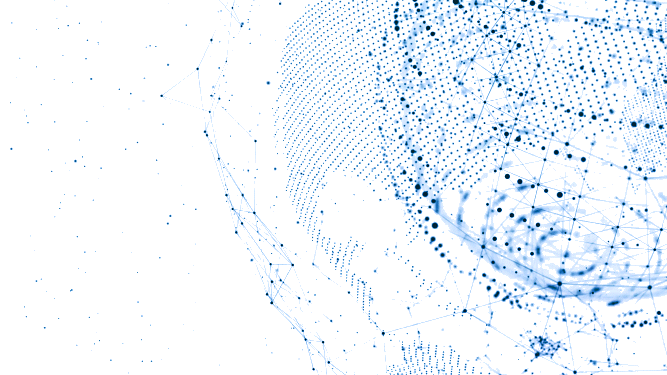